A.I. Mastered Backgammon, Chess and Go. Now It Takes On StarCraft II
DeepMind’s AlphaStar learns the complex strategy video game, which has trillions and trillions of possible moves conducted in real time
/https://tf-cmsv2-smithsonianmag-media.s3.amazonaws.com/filer/0e/7b/0e7b579b-c23b-4f7e-bd23-4149be0af348/ezgifcom-resize_1.png)
Last January, during a livestream on YouTube and Twitch, professional StarCraft II player Grzegorz “MaNa” Komincz from Poland struck a blow for humankind when he defeated a multi-million-dollar artificial intelligence agent known as AlphaStar, designed specifically to pummel human players in the popular real-time strategy game.
The public loss in front of tens of thousands of eSports fans was a blow for Google parent company Alphabet’s London-based artificial intelligence subsidiary, DeepMind, which developed AlphaStar. But even if the A.I. lost the battle, it had already won the war; a previous iteration had already defeated Komincz five times in a row and wiped the floor with his teammate, Dario “TLO” Wünsch, showing that AlphaStar had sufficiently mastered the video game, which machine learning researchers have chosen as a benchmark of A.I. progress.
In the months since, AlphaStar has only grown stronger and is now able to defeat 99.8 percent of StarCraft II players online, achieving Grandmaster rank in the game on the official site Battle.net, a feat described today in a new paper in the journal Nature.
/https://tf-cmsv2-smithsonianmag-media.s3.amazonaws.com/filer/c4/aa/c4aadf69-533c-4ca6-9e07-6242eef08f43/ezgifcom-resize_17.jpg)
Back in 1992, IBM first developed a rudimentary A.I. that learned to become a better backgammon player through trial and error. Since then, new A.I. agents have slowly but surely dominated the world of games, and the ability to master beloved human strategy games has become one of the chief ways artificial intelligence is assessed.
In 1997, IBM’s DeepBlue beat Gary Kasparov, the world’s best chess player, launching the era of digital chess supremacy. More recently, in 2016, Deepmind’s AlphaGo beat the best human players of the Chinese game Go, a complex board game with thousands of possible moves each turn that some believed A.I. would not crack for another century. Late last year, AlphaZero, the next iteration of the A.I., not only taught itself to become the best chess player in the world in just four hours, it also mastered the chess-like Japanese game Shogi in two hours as well as Go in just days.
While machines could probably dominate in games like Monopoly or Settlers of Catan, A.I. research is now moving away from classic board games to video games, which, with their combination of physical dexterity, strategy and randomness can be much harder for machines to master.
“The history of progress in artificial intelligence has been marked by milestone achievements in games. Ever since computers cracked Go, chess and poker, StarCraft has emerged by consensus as the next grand challenge,” David Silver, principal research scientist at DeepMind says in a statement. “The game’s complexity is much greater than chess, because players control hundreds of units; more complex than Go, because there are 1026 possible choices for every move; and players have less information about their opponents than in poker.”
David Churchill, a computer scientist at the Memorial University of Newfoundland who has run an annual StarCraft A.I. tournament for the last decade and served as a reviewer for the new paper, says a game like chess plays into an A.I.’s strengths. Each player takes a turn and each one has as long as possible to consider the next move. Each move opens up a set of new moves. And each player is in command of all the information on the board—they can see what their opponent is doing and anticipate their next moves.
“StarCraft completely flips all of that. Instead of alternate move, it’s simultaneous move,” Churchill says. “And there’s a ‘fog of war’ over the map. There’s a lot going on at your opponent’s base that you can’t see until you have scouted a location. There’s a lot of strategy that goes into thinking about what your opponent could have, what they couldn’t have and what you should do to counteract that when you can’t actually see what's happening.”
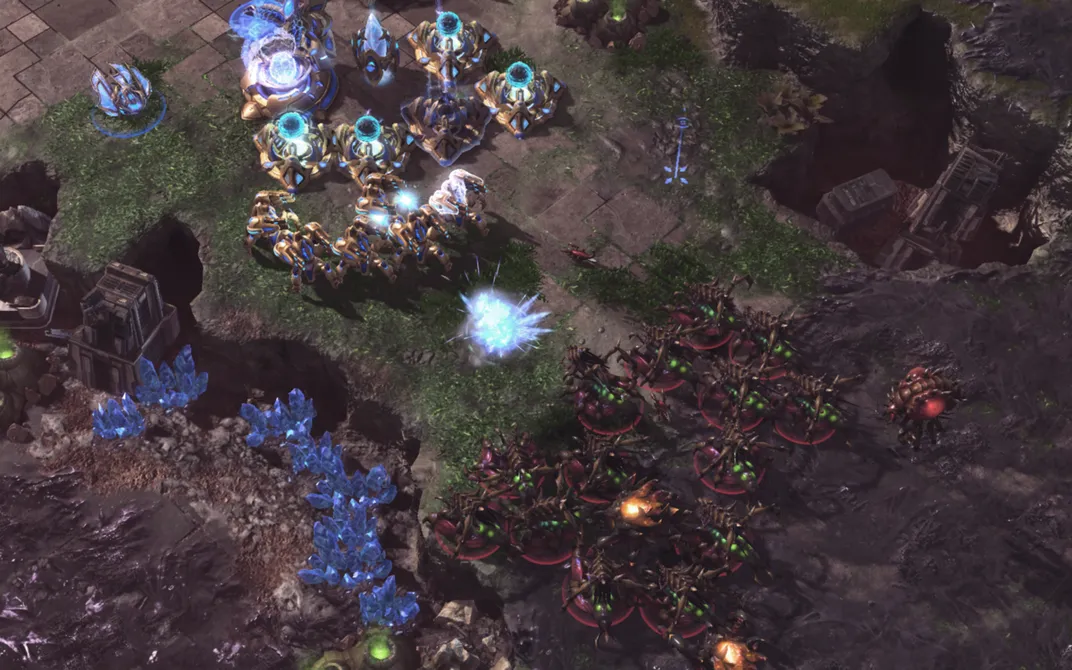
Add to that the fact that there can be 200 individual units on the field at any given time in StarCraft II, each with hundreds of possible actions, and the variables become astronomical. “It’s a way more complex game,” Churchill says. “It’s almost like playing chess while playing soccer.”
Over the years, Churchill has seen A.I. programs that could master one or two elements of StarCraft fairly well, but nothing could really pull it all together. The most impressive part of AlphaStar, he says, isn’t that it can beat humans; it’s that it can tackle the game as a whole.
So how did DeepMind’s A.I. go from knocking over knights and rooks to mastering soccer-chess with laser guns? Earlier A.I. agents, including DeepMind’s FTW algorithm which earlier this year studied teamwork while playing the video game Doom III, learned to master games by playing against versions of themselves. However, the two machine opponents were equally matched and equally aggressive algorithms. Because of that, the A.I. only learned a few styles of gameplay. It was like matching Babe Ruth against Babe Ruth; the A.I. learned how to handle home runs, but had less success against singles, pop flies and bunts.
The DeepMind team decided that for AlphaStar, instead of simply learning by playing against high-powered versions of itself, it would train against a group of A.I. systems they dubbed the League. While some of the opponents in the League were hell-bent on winning the game, others were more willing to take a walloping to help expose weaknesses in AlphaStar’s strategies, like a practice squad helping a quarterback work out plays.
That strategy, combined with other A.I. research techniques like imitation learning, in which AlphaStar analyzed tens of thousands of previous matches, appears to work, at least when it comes to video games.
Eventually, DeepMind believes this type of A.I. learning could be used for projects like robotics, medicine and in self-driving cars. “AlphaStar advances our understanding of A.I. in several key ways: multi-agent training in a competitive league can lead to great performance in highly complex environments, and imitation learning alone can achieve better results than we’d previously supposed,” Oriol Vinyals, DeepMind research scientist and lead author of the new paper says in a statement. “I’m excited to begin exploring ways we can apply these techniques to real-world challenges.”
While AlphaStar is an incredible advance in AI, Churchill thinks it still has room for improvement. For one thing, he thinks there are still humans out there that could beat the AlphaStar program, especially since the A.I. needs to train on any new maps added to the game, something he says human players can adapt to much more quickly. “They’re at the point where they’ve beaten sort of low-tier professional human players. They’re essentially beating benchwarmers in the NBA,” he says. “They have a long way to go before they’re ready to take on the LeBron James of StarCraft.”
Time will tell if DeepMind will develop more techniques that make AlphaStar even better at blasting digital aliens. In the meantime, the company’s various machine learning projects have been challenging themselves against more earthly problems like figuring out how to fold proteins, decipher ancient Greek texts, and learning how to diagnose eye diseases as well or better than doctors.