Scientists Used an Ordinary Digital Camera to Peer Around a Corner
A team from Boston University recreated an image of an object using its shadow
/https://tf-cmsv2-smithsonianmag-media.s3.amazonaws.com/filer/88/4b/884b8482-ac7b-4d3c-9ac9-e4f837153b0d/screen_shot_2019-01-23_at_22951_pm.png)
You don’t need superpowers to see what’s hiding around the corner; All you need is the right algorithms, basic computing software and an ordinary digital camera, a team of researchers show in a paper published today in Nature.
Inventing efficient ways to spot objects outside a human’s line of sight is a common goal for scientists studying anything from self-driving cars to military equipment. In its simplest form, this can be done using a periscope, which is a tube with multiple mirrors that redirect light. Previous efforts to bring this brick-and-mortar device into the digital era involved using sensitive, high-tech equipment to measure the time it takes for light to hit a sensor, allowing researchers to approximate the hidden object’s relative position, size and shape. While these techniques get the job done, it’s difficult to apply to everyday use because of its cost and complexity, notes the new study’s lead author Vivek Goyal, an electrical engineer at Boston University.
Previous studies had shown that an ordinary digital camera could be used to recreate 1-D images of out-of-sight objects. Goyal and his team decided to expand on that technique and create 2-D images.
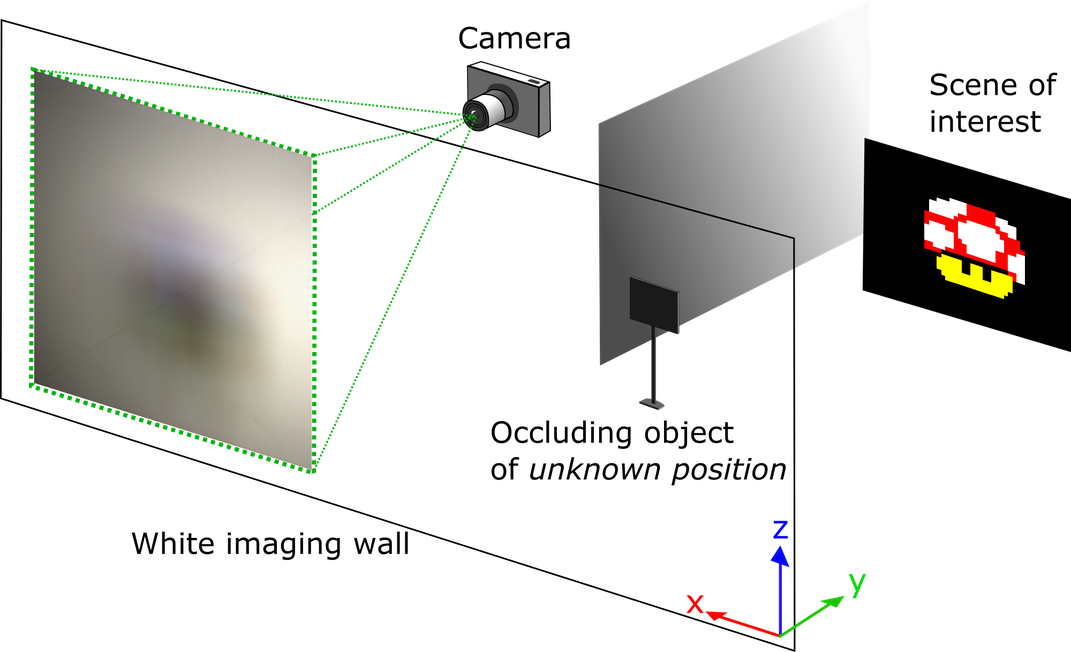
The experiment worked like this: The team pointed a digital camera at a white wall. Then, around a corner sitting parallel to the camera, they positioned an LCD screen to face the same white wall. The screen displayed a simple 2-D image—in this case, a Nintendo mushroom, a yellow emoticon with a red sideways hat or the letters BU (for Boston University) in big, bold red font. The white wall functioned like a mirror in a periscope. By using long exposure when taking a picture with the camera, the team captured the soft blur of light glowing on the white wall from the screen.
However, there’s a reason that a white wall looks white, Goyal says. Unlike a mirror—which reflects light in a specific direction—a wall scatters reflected light in all different angles, rendering any recreated image an unintelligible mess of pixelated colors to the naked eye. Surprisingly, it’s easier to recreate the hidden image when there’s something blocking it, also called an occluding object.
The occluding object—for this study, a chair-like panel—allowed the team to recreate an image using the science of penumbri, an everyday phenomenon created when light casts partial shadows in a sort of halo around an opaque object.
“Penumbri are everywhere,” Goyal says. “[If] you’re sitting somewhere with overhead fluorescent lighting, because your lighting is not from a single point, objects aren’t casting sharp shadows. If you hold your hand out...you see a bunch of partial shadows instead of complete shadowing.” In essence, those partial shadows are all penumbra.
So, even though the occluding object blocked part of the picture, the shadows provided the algorithm with more data to use. From there, reversing the path of the light just required simple physics.
It likely sounds illogical and complicated, but electrical engineer Genevieve Gariepy, who studied non-line-of-sight imaging while completing her PhD at Heriot-Watt in Edinburgh, described it as a high-tech game of 20 questions. Essentially, the occluding object in this experiment functions in the same way a good question would in the game.
“The inverse problem in [20 questions is] guessing who I’m [thinking] about,” she explains. “If we play the game and I think about...let’s say Donna Strickland, who just won the Nobel Prize in Physics. If you ask me ‘Is she a woman? Is she alive?’ it’s very complicated because [those descriptions could apply to] so many people. If you ask me ‘Did she win a Nobel Prize?’ then it becomes much easier to guess who I’m thinking about.”
The initial measurements look like blurry black blobs, so Goyal and his team were far from certain their technique would produce a clear image. “We were sure that something was possible, [but it could have been] really, really terrible in quality,” Goyal says.
So, when the first recreation came through in strong detail, it was “a great, pleasant surprise,” says Goyal. Though the image is far from perfect, letters are readable, colors are clear, and even the yellow emoticon’s face was identifiable. The team was able to obtain the same level of accuracy when working with simple video.
Goyal is most excited about the accessible nature of this technology. “Our technique [uses] conventional hardware,” he says. “You could imagine that we could write an app for a mobile phone that does this imaging. The type of camera we’ve used is not fundamentally different from a mobile phone camera.”
Both Goyal and Gariepy agree one of the most likely future uses of this technology would be in autonomous vehicles. Currently, those vehicles have humans beat by being able to sense what’s directly around them on all sides, but the range of those sensors doesn’t exceed the average human field-of-view. Incorporating this new technology could take cars to the next level.
“You could imagine [a car] being able to sense that there’s a child on the other side of a parked car, or to be able to sense as you approach an intersection in an urban canyon that there’s cross-traffic coming that’s not in your line of sight,” Goyal says. “It’s an optimistic vision, but not unreasonable.”