A Startup Wants to Track Everything From Shoppers to Corn Yields Using Satellite Imagery
Orbital Insight, founded by a NASA and Google veteran, is quick to predict crop failures and estimate the current global oil surplus
/https://tf-cmsv2-smithsonianmag-media.s3.amazonaws.com/filer/e0/ac/e0ac0bc9-5a98-4cb5-812d-08397bff7aee/tanks_montage.jpg)
Big data is getting so big, it’s slipping the surly bonds of Earth.
A startup called Orbital Insight, which recently raised nearly $9 million in funding, is using satellite imagery and cutting-edge computing techniques to estimate global oil surplus, predict crop shortfalls before harvest time and spot retail trends by keeping track of the number of cars in big-box parking lots. It should also be possible to train the software to spot illegal deforestation early and better track climate change.
The company uses machine learning techniques and computing networks that mimic the human brain to spot patterns in massive amounts of visual data. Facebook uses similar techniques to recognize faces in uploaded images and auto-tag you and your friends. But instead of searching for faces, Orbital Insight is taking advantage of the growing abundance of satellite imagery, thanks to the rise of small, low-cost satellites, and teaching their networks to automatically recognize things like vehicles, the rate of construction in China and the shadows cast by floating-lid oil containers, which change depending on how full they are.
It would be impossible, of course, for humans to sift through regularly updated global satellite imagery. But with massively parallel computers and advanced pattern-recognition techniques, Orbital Insight aims to deliver types of data that haven’t available before. Current global oil estimates, for instance, are already six weeks old when they’re published. With Orbital, analysis of crop yields could be delivered mid-season—important information to have, whether you’re a high-level United Nations worker trying to get ahead of a food crisis, or a commodities trader working for a hedge fund.
Orbital Insight hasn’t been around long—it was founded in late 2013 and only came out of “stealth mode” late last year. But the company’s founder, James Crawford, has plenty of experience in compatible fields. A former autonomy and robotics head at NASA’s Ames Research Center, he also spent two years as engineering director at Google Books, turning archived printed pages into searchable text.
Several companies, like Spire and Inmarsat, and even Tesla’s Elon Musk, are working on hardware—designing and launching new networks of satellites—but Crawford says Orbital Insight is instead focusing purely on software.
“In some ways I see what we’re doing here in the impetus of this company,” says Crawford, “is taking a lot of the learning [at Google] about how to do big data, how to apply [artificial intelligence], how to apply machine learning to these pipelines of images, and apply that to the satellite space. ”
Crawford’s company may be one of the few working on using emerging software techniques such as artificial neural networks and machine learning to parse satellite imagery. But the technique he's using, also known as deep learning, is exploding in the technology space at the moment. Established companies like Facebook, Google and Microsoft are using deep learning techniques for things like automatic image tagging and improved speech recognition and translation. IBM also recently acquired a deep learning company, called AlchemyAPI, to enhance their Watson computer system.
With deep learning, powerful computers and multiple layers of concurrently running pattern recognition (hence the "deep" in deep learning) mimic the neural networks of the human brain. The aim is to get a computer to “learn” to recognize patterns or perform tasks that would be too complex and time-consuming to “teach” using traditional software.
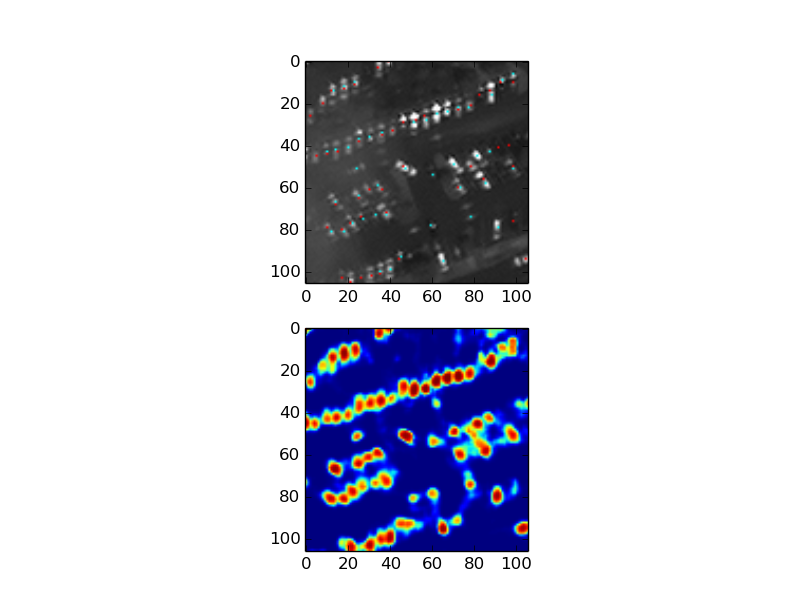
The details of deep learning are technical, but at the very basic level, it’s surprisingly simple. When it comes to measuring retail trends with parking lot activity, Crawford says the company first has employees manually mark cars in a few hundred parking lots with red dots. “Then, you feed each individual car into the neural network, and it generalizes the patterns of light and dark, the pattern of pixels of a car,” says Crawford. “And when [the computer] looks at a new image, what it’s essentially doing is fairly sophisticated, but still basically a pattern match.”
When estimating retail activity, Crawford says his company is much better at inferring how a chain is doing on a national level, by measuring how full parking lots are over time and comparing that to how full the same lots were in previous quarters using older images, than gauging the health of an individual store.
He admits that many retailers already have ways of tracking this data for their own stores, but they would be happy to know how their competitors are doing months before financial results are released. The same would be true of hedge funds, who Crawford says are some of the company’s earliest customers. It’s easy to see how this kind of data could give investors a leg up. The satellite imagery is already available, and Orbital Insight is just parsing it, so it’s unlikely to spark any insider trading concerns.
If the network makes an occasional mistake, say confusing a dumpster for a car, it’s not much of a problem, Crawford explains, because the mistakes tend to cancel each other out on a large scale. For things like oil estimates, even if they’re off by several percentage points, it’s still better than waiting up to six weeks for more concrete data.
While the startup seems focused on providing data to market investors first, what the company does could be put to more altruistic uses as well. “We’re curious in the future about using this to detect deforestation, and to detect things like road building that could be a precursor to deforestation,” says Crawford. “There’s also really interesting things that can be done around looking at snow pack, water and other aspects for climate change.” He also says they’re looking into third-world agriculture, and says multi-spectral imagery is a good way to tell how healthy plants are, to predict crop failures.
Of course, any aspect of big data that also incorporates satellite imagery brings up privacy issues. But Orbital Insight isn’t taking the photos, they’re accessing and analyzing images that are already available. And as Crawford points out, current U.S. regulations for commercial imaging satellites stipulate that you can’t go below 20 cm per pixel. At that resolution, the average person would show up as a few dots. So it would be tough to distinguish individual people at all, let alone a person’s identity or even gender.
Crawford says that much of the short-term advances in deep learning techniques in general will involve simplifying and automating the tweaks to the algorithms (meaning less manually tagging cars or corn fields), so that companies can more quickly apply machine learning to new areas.
As for the future of Orbital Insight specifically, the company's founder definitely isn’t talking small. He likens what the company is doing to creating a “macroscope” that could impact the world to a similar degree that the microscope transformed biology.
“A lot of what we’re seeing about the Earth, whether it’s corn yield or deforestation, or oil inventory, are so big that you can’t see them with the human eye because you’d have to process a million images at once,” says Crawford. “It will ultimately change the way we view the Earth, change the way we think about it, and change the way we think about managing it.”