Better Traffic-Light Timing Will Get You There Faster
New algorithms from MIT researchers keep gridlock at bay by predicting traffic before it starts
/https://tf-cmsv2-smithsonianmag-media.s3.amazonaws.com/filer/bb/13/bb136857-2588-431f-92ea-3db3091ea0ad/mitnews_lausannetraffic_olivares_01.jpg)
It happens to every driver more often than he or she would probably like: Travel along a planned route, and somehow one manages to hit every single light along the way. Not only is it frustrating, but that stop-and-go wastes fuel, time and can even cause gridlock, grinding an entire city to a halt.
New simulation software developed by Carolina Osorio, an assistant professor of civil and environmental engineering at MIT, promises to smooth traffic in a more effective way than any software before it. By optimizing the timing of traffic lights beyond the capabilities of current systems, her models have been shown to cut rush-hour travel times by 22 percent.
Traffic-light timing systems typically work in one of two ways. On a large city or regional scale, systems set light timing based on the observed traffic; these are called flow-based models. Other simulators work on a more micro scale, taking into account the actions and habits of individual drivers. These simulators act as a sort of artificial intelligence to help predict how driver behaviors and decisions might change in given traffic conditions. It’s those minute differences and individual decisions that throw flow-based models off-kilter.
“I need to account for how people will react to my changes. If the travel times increase on an arterial [road], then people might divert," Osorio explains. “Most signal-timing software looks at current or historical traffic patterns. It doesn’t take into account how travel might change.”
This problem can compound as more and more signal changes are implemented. Say, for example, you have two possible routes to commute to work: Route A and Route B. You most-often choose Route A, but one day the traffic-light timing changes, so you decide to switch to Route B. Not only has the flow of traffic changed on Route A, but those who were already taking Route B might be inclined to reconsider their options. Further complicating matters is how those changes and diversions might ripple outwards and affect the rest of the roads and intersections in the region.
The obvious solution is to run both flow-based and individualized models for all scenarios. But simulating every possible permutation of traffic flow isn’t feasible. The amount of computing power that it might take to complete such a complex simulation for an entire city would make the system cost prohibitive.
To work around that issue, without sacrificing fidelity and reliability, Osorio’s system combines the best of both worlds. It takes only the best flow-based scenarios, as identified by commonplace timing software, and runs the driver-specific simulations only on those cases.
Take, for example, an intersection that has a much heavier traffic flow north and south than it does east and west. Simpler models can reason that the traffic light should allow for more green time in the north-south lanes than in east-west ones. Then, the more-complex simulations can help assess just how long those lights should be and also predict the ripple effect of such a change.
The solution is scalable. “Let’s say I had 100 different signal timings that I wanted to test,” says Osorio. “The simpler model could give you an idea of a subset of the 100 that could have great potential. Then, we run the simulation on the subset.”
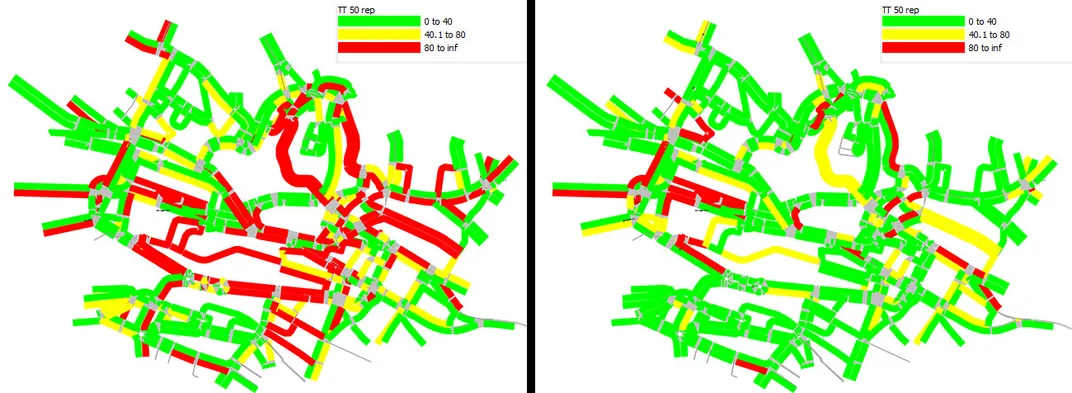
Osorio’s paper, which will be published in the journal Transportation Science, applied her model to traffic in Lausanne, Switzerland, an area where she once lived. Working with traffic data across 47 roads and 15 intersections (nine of which have traffic lights), the study applied her algorithms to the first hour of the evening rush hour. The simulations cut travel time by nearly one-quarter.
The traffic-flow simulators that Osorio feeds into her models are typically created by cities themselves. Municipalities collect their own data about prevailing traffic conditions and mine census information, among other methods, to create models that they trust. They then hand over their simulations embedded with metadata about their infrastructure, popular destinations, pedestrian traffic and other relevant priorities to Osorio.
In Manhattan, for instance, there are particular restrictions on how long pedestrians should have the right of way. The New York City Department of Transportation is already collaborating with Osorio’s team to manage flow during peak periods in high-traffic areas in Manhattan.
“Such a model can validate our active traffic-management system in Manhattan, and allow us to fine-tune our processes and improve the network operation,” Mohamad Talas, a deputy director of system engineering for the NYC DOT told MIT News.
Depending on a city’s goals, says Osorio, the model can help optimize for different factors. For example, it could time traffic to help drivers boost fuel economy.
Her team is already collaborating with companies on several projects. They are involved in an effort to help drivers of future autonomous cars identify the ideal times and places to toggle into autonomous mode in order to save fuel. Another work in progress will let car-sharing programs, such as ZipCar, better locate their pick-up and drop-off locations, so that customers will be able to more reliably estimate their trip times.
All of Osorio’s work, including the Lausanne test, is still in the simulation phase, and there’s no definite timeline for applying her traffic-timing lessons to the road.
“But that’s why we do these things,” she says, “to implement them in the real world.”